Get to know our dedicated and thriving family of Fighting Scots.
Athletics at Wooster are an integral part of our educational mission, and our principal goal is to attract prospective student-athletes who are equally committed to achieving the highest possible standard of excellence both in their athletic endeavors as well as in the classroom. Through participation and the pursuit of victory, Wooster student-athletes have the opportunity to improve their physical and mental well-being, develop leadership qualities, learn effective time-management, and develop lifelong friendships.
Our Fighting Scots have won a total of five national championships and 48 conference championships in just the past decade. They have earned an incredible 533 All-American recognitions, including 46 in the past decade. Fifty-one student-athletes have earned CoSIDA Academic All-American status and 19 have received NCAA postgraduate scholarships, including two in the 2019-20 academic year.
Our Fighting Scots have won a total of five national championships and 48 conference championships in just the past decade. They have earned an incredible 533 All-American recognitions, including 46 in the past decade. Fifty-one student-athletes have earned CoSIDA Academic All-American status and 19 have received NCAA postgraduate scholarships, including two in the 2019-20 academic year.
If you are interested in learning more about competing in intercollegiate athletics and becoming part of Wooster’s winning tradition, please complete this form and select your program(s) of interest.
Go Scots!
Amy Heasley Williams
Athletic Director
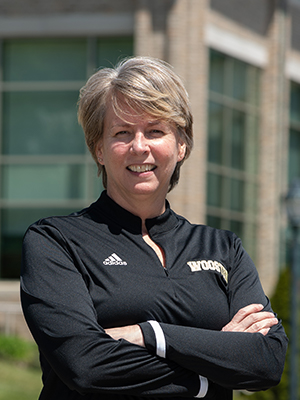
View our Scot Center, home to Fighting Scots Athletics.
Learn more about the Loud MacLeod on football Saturdays.